“AI can be a resource and help to reduce risk, but this assumes that the companies understand and actively follow up the hazards when new technology is developed and adopted,” says Linn Iren Vestly Bergh.
A senior adviser at Havtil, she heads its follow-up of the industry’s work in this field.
Different
“Many of the risks associated with AI differ from those we have experience of from the petroleum sector and conventional IT systems,” Bergh explains, and cites machine learning (ML) as an example.
“This is a key component in many AI systems. But how well ML functions depends on both the quality of the data used to train it and the method applied for this process.”
Lack of accurate training data might mean the end results put users on the wrong track, she points out. “It’s not always easy to detect such errors, particularly in complex systems.
“Weaknesses in data for and training of the ML model could also cause it to fail to recognise rare or unusual circumstances. That can create problems in reacting correctly when an unfamiliar event occurs.”
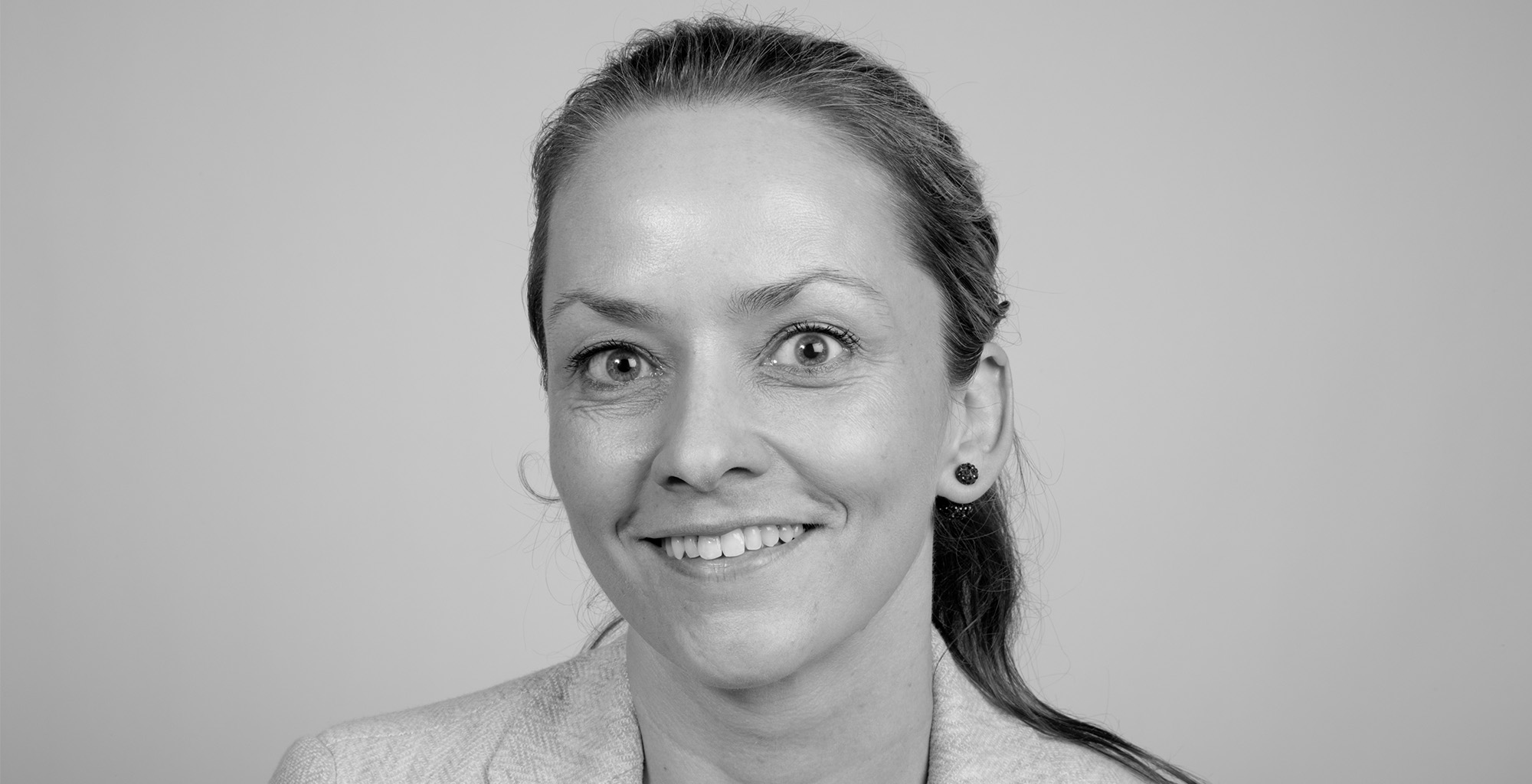
Early
“We see examples of AI being applied in the industry, and expect this to rise in coming years,” says Bergh. “But utilising it in different operations and products is at an early stage, with a high level of testing and product development.”
She cites condition monitoring and maintenance planning, autonomous cranes, automated drilling, and security of ICT systems as areas where AI is being developed and tested as part of solutions.
As AI progresses and is adopted in operations with safety significance, securing acceptable data quality and management, maintenance, meaningful human control, openness and transparency will become ever more important. That applies particularly to sectors like petroleum which involve major accident risk.
Havtil is also seeing a desire to use fully developed components or complete products even when these have often been developed for other industries or purposes. Cutting costs and simplifying integration in existing digital ecosystems are drivers for this approach.
“It’s the companies which have to own and control the risk when introducing new systems and technology,” Bergh emphasises.
“That also applies when procuring products and solutions. The companies are responsible for following up prudent development and application of AI systems. This calls for a multidisciplinary approach and internal interaction in both companies and the industry.”
Factors
AI systems also present other risk factors which may be significant for the threat of major accidents. Examples include lack of transparency and weak user interfaces as well as complexity and inadequate documentation giving poor traceability.
AI can also be misused, creating possible vulnerabilities to deliberate attack.
Bergh emphasises that good technology development is also about people, and says that AI must be viewed from an integrated perspective.
“This will allow factors associated with humans, technology and organisation (HTO) to be incorporated in developing, using and maintaining the solutions.
“Handling and assessing vulnerabilities and risk must also take account of human possibilities and limitations.”
It’s the companies which have to own and control the risk when introducing new systems and technology.
Evaluation
Offshore workers have largely moved from acquiring information in the field with the aid of eyes, ears, nose, and manual measurements and calculations to evaluating predictions presented on a screen, usually based on an ML system.
“Digital solutions can produce good and precise results,” says Bergh. “But the underlying systems may be so complex that people have difficulty understanding them.”
That opens the way to new sources of error – and increased risk. Some computer models are so complicated that decision-makers fail to grasp why particular recommendations are made, an issue often termed the black-box problem.
It can also be difficult subsequently to explain the results presented by complex models. Comprehending decision processes in models based on neural networks can be demanding, for example.
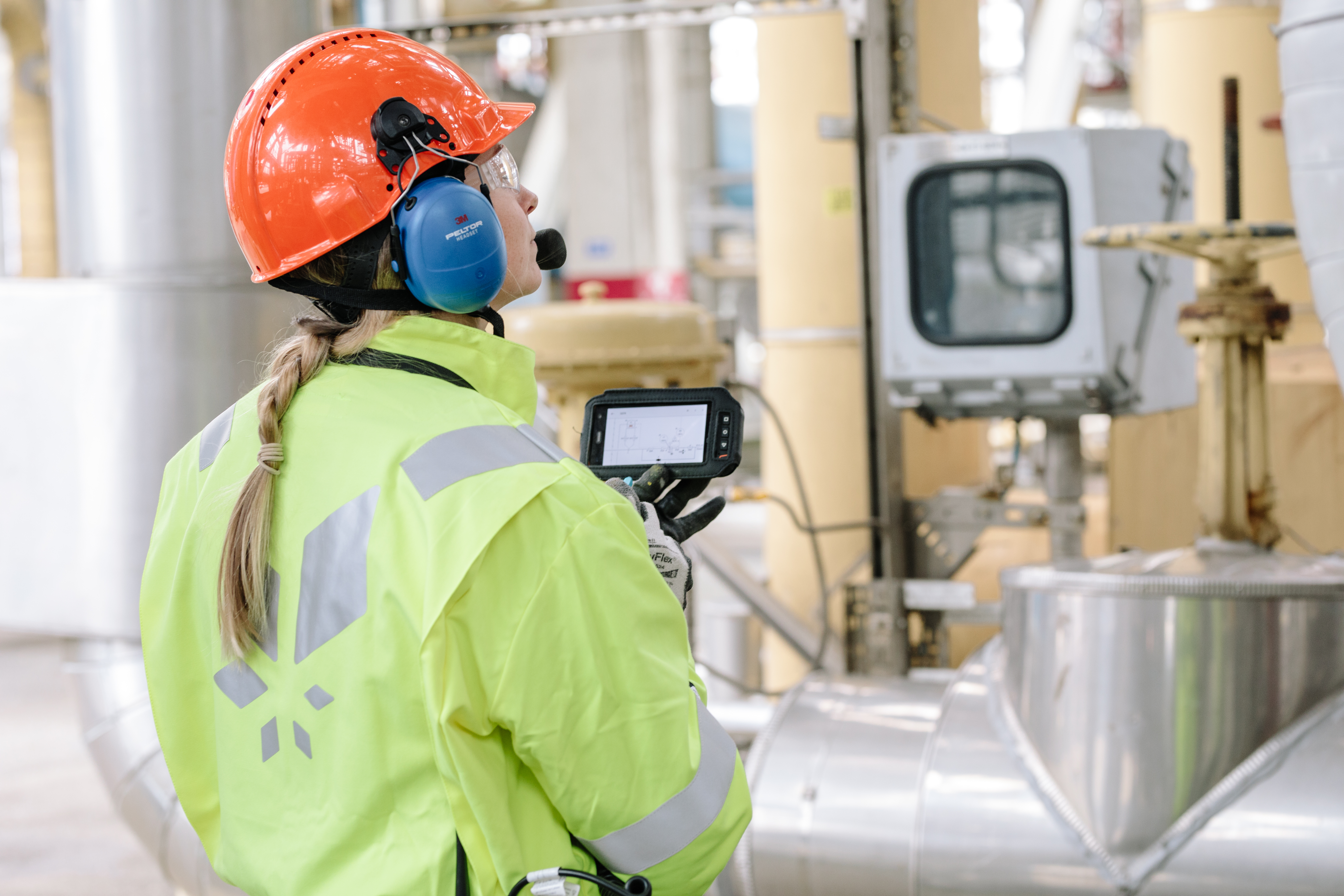
“Even though tools to inspect such models are being developed, we believe that devoting enough resources for continued development of tools and methods for inspection and risk management is crucial,” says Bergh.
“We see that the companies use AI solutions as decision support, where people still have a hand on the wheel. But a danger also exists that the supervising person’s attention wanders – partly because the work becomes routine or the ‘truth’ presented by the AI system clouds their judgement.”
Podcast: The 5 Paradoxes of AI
In this episode of the Havtil podcast, we talk to Mica Endsley, director of SA Technologies and former chief scientist for the US Air Force. She has distinguished herself as a leading scientist within responsible artificial intelligence, and here she gives us an insight into what she calls the 5 paradoxes of AI.
Monitoring
Human error often occurs because a gap exists between technological and human traits. Introducing AI will increasingly reduce personnel to a supervisory and passive role.
That creates a need for vigilance – in other words, the ability to detect errors and react quickly if abnormal circumstances occur. Experience shows that simple monitoring fails to make optimum use of people’s intrinsic strengths.
“Training and education are key, but the system must be designed with humans at the centre,” Bergh observes.
“That’s an important issue which we highlight in our audits, but one which experts are also raising to a great extent as the technology becomes more complex.”
Increased attention
Havtil will be devoting increased attention during coming years to safety-related aspects of AI in the petroleum sector.
“Our ambition is to ensure that safe and beneficial operating parameters are established for AI use while actively following up that the industry manages risk factors related to developing and maintaining such solutions,” affirms Bergh.
The authority will work in the next few years to increase knowledge about risk factors associated with using AI in operations of significance for offshore safety.
A key place will be given in this work to the uncertainty related to the actual AI models and best practice for developing safe and reliable AI solutions.
Training and education are key, but the system must be designed with humans at the centre.
Meetings
A number of meetings were held by Havtil with petroleum-sector operators and suppliers in 2023 to secure information about their work with AI.
“These sessions showed that the industry has ambitious goals for applying this technology to improve efficiency and safety,” says Bergh.
“Risk management of AI is an immature field in general, and that’s reflected in our industry. We see the players have started work on this, but that it’s in an early phase.”
It emerged from the meetings that the companies are making efforts to establish appropriate and systematic methods for identifying and managing AI-related risk.
However, practice for developing and maintaining such systems is less established. As a result, the guidelines for managing the systems are not in place either.
“Although applying AI is in a start-up phase, we see that it’s already being used in planning and decision-support systems,” Bergh notes. “So it’ll be important to look at how the companies can utilise the technology in the safest possible way.
“Another important goal will be to strengthen our enforcement powers through regulation, audits and advice on following-up AI in the industry.”
HSE regulations
The health, safety and environmental regulations for Norway’s petroleum sector are performance-based, technologically neutral and built on risk-management principles.
“Regulation can play a key role in innovation by providing stable and predictable operating parameters for companies in the industry with regard to both development and application,” says Bergh.
Havtil is now taking a closer look at the legal challenges posed by using AI in the petroleum sector. These include the relationship between principles and requirements in the HSE regulations and AI’s unique characteristics.
“Our assessment so far is that the HSE regulations specify a number of requirements which also apply to the use of AI,” explains Bergh.
“However, risk factors could exist which will require further development of the regulations and their associated guidance.
“References to norms and standards for AI can be included in the guidance section as and when required.
“Over time, such references might cover existing and forthcoming standards, recommendations and compliance assessments, which is in line with the way the regulations already function.”
Prudent
“The question in the future will be less about whether we’re going to adopt AI and more about how we do this in a prudent way,” Bergh concludes.
“It’s important for this work that employers, employees and government collaborate, engage with it, and contribute experience and expertise.”